|
1. 综述
基于遥感数据的变化检测是探测地表变化的一种重要方法,在城市规划、环境监测、农业调查、灾害评估、地图修改等方面有着广泛的应用。近年来,集成人工智能(AI)技术成为开发新的变化检测方法的研究热点。尽管一些研究人员声称基于人工智能的变更检测方法优于传统的变更检测方法,但人工智能如何以及在多大程度上提高变更检测性能尚不明显。本文综述了人工智能在变化检测中的最新方法、应用和挑战。
具体来说,首先介绍了基于AI的变化检测的实现过程。然后,介绍了用于变化检测的不同传感器的数据,包括光学遥感数据、合成孔径雷达(SAR)数据、街景图像和组合异构数据,并列出了可用的开放数据集。系统地回顾和分析了基于人工智能的变化检测方法的一般框架,并进一步分析了基于人工智能的变化检测中使用的无监督方案。随后描述了AI中常用的用于变化检测的网络。从实际应用的角度出发,根据其适用性对基于AI的变化检测方法的应用领域进行了分类。
最后,讨论和描述了AI在变化检测方面的主要挑战和前景,包括(a)异构大数据处理,(b)无监督AI,(c)AI的可靠性。这一综述将有助于研究者对这一领域的认识。
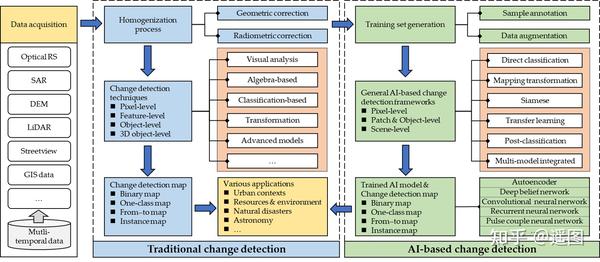
图1。变更检测通用原理图。
2. 实施过程
图2给出了基于AI的变更检测的一般实现过程,但是AI模型的结构是多样的,需要根据不同的应用情况和训练数据进行很好的设计。值得一提的是TensorFlow, Keras, Pytorch, 和 Caffe, 等现有成熟框架帮助研究人员更轻松地实现AI模型的设计、训练和部署,其开发文档提供了详细的介绍。
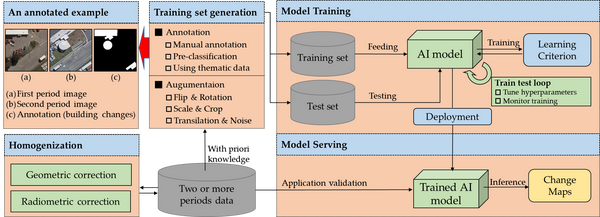
图2。基于AI的变更检测实现过程(黑色箭头表示工作流程,红色箭头表示实例)。
2.1 基于AI的方法的可用代码
表1。基于AI的变化检测方法的可用代码列表。
方法 | 关键词 | 论文/代码 | (Re-)框架 | SRCDNet | CNN; Siamese; Attention; Super-resolution; Optical RS | 针对不同分辨率图像的基于叠加注意模块的超分辨率变化检测网络,TGRS,2021. [paper](https://doi.org/10.1109/TGRS.2021.3091758), [code, dataset](https://github.com/liumency/SRCDNet) | Pytorch 1.2 | ESCNet | CNN; Siamese; Superpixel; Optical RS | 一种用于甚高分辨率遥感图像的端到端超像素增强变化检测网络。TNNLS,2021年。 [paper](https://doi.org/10.1109/TNNLS.2021.3089332), [code](https://github.com/Bobholamovic/ESCNet) | Pytorch 1.3 | KPCAMNet | CNN; Siamese; KPCA; Unsupervised; Optical RS | 基于深核PCA卷积映射网络的多时相VHR图像无监督变化检测,TCYB,2021。 [paper](https://doi.org/10.1109/TCYB.2021.3086884), [code](https://github.com/I-Hope-Peace/KPCAMNet) | Python | SeCo | CNN (ResNet); Transfer Learning; Optical RS | 对比:来自非策展遥感数据的非监督预训练,arXiv,2021 [paper](https://arxiv.org/abs/2103.16607), [code, dataset](https://github.com/ElementAI/seasonal-contrast) | Pytorch 1.7 | CapsNet | Capsule Network(SegCaps); CVA; Siamese; Optical RS | 对比:来自非策展遥感数据的非监督预训练,arXiv,2021 [paper 1](https://doi.org/10.1109/LGRS.2020.3022512), 用于光学遥感影像探测的改变网络,RS,2021. [paper 2](https://doi.org/10.1109/LGRS.2020.3022512), [code, dataset](https://github.com/xuquanfu/capsule_change_detection) | Keras | BIT_CD | CNN (ResNet18); Siamese; Attention; Transformer; Optical RS | 利用变换进行遥感影像变化检测,TGRS,2021。 [paper](https://doi.org/10.1109/TGRS.2021.3095166), [code, dataset, pre-trained model](https://github.com/justchenhao/BIT_CD) | Pytorch 1.6 | IAug_CDNet | CNN (GauGAN+UNet); Siamese; GAN; Supervised; Optical RS | 遥感图像中建筑物变化检测的对抗性实例增强,TGRS,2021. [paper](https://doi.org/10.1109/TGRS.2021.3066802), [code, dataset](https://github.com/justchenhao/IAug_CDNet) | Pytorch | DDNet | CNN; DI+FCM; Unsupervised; SAR | 使用双域网络的合成孔径雷达图像变化检测,GRSL,2021。 [paper](https://doi.org/10.1109/LGRS.2021.3073900), [code, dataset](https://github.com/summitgao/SAR_CD_DDNet) | Pytorch | SNUNet-CD | CNN (NestedUNet); Siamese; Attention; Supervised; Optical RS | SNUNET-CD:用于VHR图像变化检测的密集连接暹罗网络,GRSL,2021年。 [paper](https://doi.org/10.1109/LGRS.2021.3056416), [code, dataset, pre-trained model](https://github.com/likyoo/Siam-NestedUNet) | Pytorch 1.4 | DSMSCN | CNN; Siamese; Multi-scale; Unsupervised/Supervised; Optical RS | 用于高分辨率双时相遥感图像变化检测的深度监督图像融合网络,arXiv,2020。 [paper](https://arxiv.org/abs/1906.11479), [code, dataset](https://github.com/I-Hope-Peace/DSMSCN) | Tensorflow 1.9 | SiamCRNN | CNN+RNN; Siamese; Multi-source; Optical RS | 基于深暹罗卷积多层递归神经网络的多源VHR图像变化检测,TGRS,2020。 [paper](https://doi.org/10.1109/TGRS.2019.2956756), [code, dataset](https://github.com/I-Hope-Peace/SiamCRNN) | Tensorflow 1.9 | DSIFN | CNN; Attention Mechanism; Optical RS | 用于高分辨率双时相遥感图像变化检测的深度监督图像融合网络,ISPRS,2020。 [paper](https://doi.org/10.1016/j.isprsjprs.2020.06.003), [code, dataset](https://github.com/GeoZcx/A-deeply-supervised-image-fusion-network-for-change-detection-in-remote-sensing-images) | Pytorch & Keras | CEECNet | CNN; Attention Mechanism; Similarity Measure; Optical RS | Looking for change? Roll the Dice and demand Attention, arXiv, 2020. [paper](https://arxiv.org/abs/2009.02062), [code, dataset](https://github.com/feevos/ceecnet) | MXNet + Python | LamboiseNet | CNN (Light UNet++); Optical RS | 使用深度学习的卫星图像变化检测,硕士论文。 [code, dataset, pre-trained model](https://github.com/hbaudhuin/LamboiseNet) | Pytorch | DTCDSCN | CNN; Siamese | 使用双重任务约束的深暹罗卷积网络模型建立遥感图像的变化检测,进行综述。 [code, dataset](https://github.com/fitzpchao/DTCDSCN) | Pytorch | Land-Cover-Analysis | CNN (UNet); Post-Classification; Optical RS | 利用卷积神经网络检测气旋影响地区的土地利用/土地覆盖变化。 [report](https://github.com/Kalit31/Land-Cover-Analysis/blob/master/Report.pdf), [code, dataset, pre-trained model](https://github.com/Kalit31/Land-Cover-Analysis) | TensorFlow+Keras | CorrFusionNet | CNN; Scene-level; Siamese; Optical RS | 基于相关的融合网络在多时相场景分类和变化检测方面的研究进展。 [code, pre-trained model](https://github.com/rulixiang/CorrFusionNet), [dataset](https://github.com/rulixiang/MtS-WH-Dataset) | TensorFlow 1.8 | SSCDNet | CNN (ResNet18); Siamese; Transfer Learning; Semantic; Streetview | 基于弱监督剪影的语义场景变化检测,ICRA,2020。 [paper](https://arxiv.org/abs/1811.11985)
[code, dataset, pre-trained model](https://github.com/xdspacelab/sscdnet) | Pytorch+Python3.6 | Heterogeneous_CD | AE (Code-Aligned AE); Unsupervised; Transformation; Heterogeneous; Optical RS | 用于多模态遥感图像无监督变化检测的代码对齐自动编码器,arXiv,2020。 [paper](https://arxiv.org/abs/2004.07011)
[code, dataset](https://github.com/llu025/Heterogeneous_CD/tree/master/Code-Aligned_Autoencoders) | TensorFlow 2.0 | FDCNN | CNN (VGG16); Transfer Learning; Pure-Siamese; Multi-scale; Optical RS | 一种基于特征差分卷积神经网络的变化检测方法,TGRS,2020。 [paper](https://dx.doi.org/10.1109/tgrs.2020.2981051)
[code, dataset, pre-trained model](https://github.com/MinZHANG-WHU/FDCNN) | Caffe+Python2.7 | STANet | CNN (ResNet-18); Attention Mechanism; Pure-Siamese; Spatial–Temporal Dependency; Optical RS | 基于时空注意力的遥感影像变化检测方法及新数据集,RS,2020。 [paper](https://dx.doi.org/10.3390/rs12101662)
[code, dataset](https://github.com/justchenhao/STANet) | Pytorch+Python3.6 | X-Net | CNN; Unsupervised; Transformation; Heterogeneous; Optical RS; SAR | 面向无监督多模态变化检测的基于亲和力变化先验的深度图像翻译,2020。 [paper](https://arxiv.org/abs/2001.04271)
[code, dataset](https://github.com/llu025/Heterogeneous_CD/tree/master/legacy/Deep_Image_Translation) | Tensorflow 1.4 | ACE-Net | AE (Adversarial Cyclic Encoders); Unsupervised; Transformation; Heterogeneous; Optical RS; SAR | 面向无监督多模态变化检测的基于亲和力变化先验的深度图像翻译,2020。 [paper](https://arxiv.org/abs/2001.04271)
[code, dataset](https://github.com/llu025/Heterogeneous_CD/tree/master/legacy/Deep_Image_Translation) | Tensorflow 1.4 | VGG_LR | CNN (VGG16); Transfer Learning; Pure-Siamese; SLIC; Low Ranks; Optical RS | 基于深度特征和低秩的变化检测,GRSL,2017。 [paper](https://dx.doi.org/10.1109/LGRS.2017.2766840)
[re-implementation code, dataset, pre-trained model](https://github.com/MinZHANG-WHU/FDCNN/tree/master/vgg_lr) | Caffe+Matlab | CDNet | CNN; Siamese; Multimodal Data; Point Cloud Data | 在机载激光扫描和摄影测量数据之间检测建筑变化,RS,2019。 [paper](https://doi.org/10.3390/rs11202417), [code](https://github.com/Zhenchaolibrary/PointCloud2PointCloud-Change-Detection) | Pytorch | SCCN | AE (DAE); Unsupervised; Heterogeneous; Optical RS; SAR | 基于异构光学和雷达图像的变化检测深度卷积耦合网络,TNNLS,2018。 [paper](https://dx.doi.org/10.1109/TNNLS.2016.2636227)
[re-implementation code](https://github.com/llu025/Heterogeneous_CD/tree/master/Code-Aligned_Autoencoders) | TensorFlow 2.0 | cGAN | GAN (conditional GAN); Heterogeneous; Optical RS; SAR | 异构图像变化检测的条件对抗网络,GRSL,2019。 [paper](https://dx.doi.org/10.1109/LGRS.2018.2868704)
[re-implementation code](https://github.com/llu025/Heterogeneous_CD/tree/master/Code-Aligned_Autoencoders) | TensorFlow 2.0 | DASNet | CNN (VGG16); Siamese; Attention Mechanism ; Optical RS | DASNet:用于高分辨率卫星图像变化检测的双关注全卷积暹罗网络,arXiv,2020。 paper
[code, dataset, pre-trained model](https://github.com/lehaifeng/DASNet) | Pytorch+Python3.6 | UNetLSTM | CNN (UNet); RNN (LSTM); Integrated Model; Optical RS | 从多时相Sentinel-2数据用递归神经网络检测城市变化,IGARSS,2019. [paper](https://arxiv.org/abs/1910.07778)
[code, dataset, pre-trained model](https://github.com/granularai/chip_segmentation_fabric) and [code](https://github.com/SebastianHafner/urban_change_detection) | Pytorch+Python3.6 | CDMI-Net | CNN (Unet); Pure-Siamese; Multiple Instance Learning; Landslide Mapping; Optical RS | 滑坡制图的深度多实例学习,GRSL,2020。 [paper](https://dx.doi.org/10.1109/LGRS.2020.3007183)
[code, pre-trained model](https://github.com/MinZHANG-WHU/CDMI-Net) | Pytorch+Python3.6 | DSFANet | DNN; Unsupervised; Pre-classification; Slow Feature Analysis; Optical RS | 多时相遥感影像变化检测的无监督深度慢特征分析,TGRS,2019。 [paper](https://dx.doi.org/10.1109/TGRS.2019.2930682)
[code, dataset](https://github.com/rulixiang/DSFANet) | TensorFlow 1.7 | CD-UNet++ | CNN (improved UNet++); Direct Classification; Optical RS | 使用改进的UNET++对高分辨率卫星图像进行端到端变化检测,RS,2019。 [paper](https://doi.org/10.3390/rs11111382)
[code](https://github.com/daifeng2016/End-to-end-CD-for-VHR-satellite-image) | TensorFlow+Keras | SiameseNet | CNN (VGG16); Pure-Siamese; Optical RS | 卫星图像中基于斑块变化检测的多级特征暹罗网络,GlobalSIP,2018年。 [paper](https://sigport.org/documents/siamese-network-multi-level-features-patch-based-change-detection-satellite-imagery)
[code, dataset](https://github.com/vbhavank/Siamese-neural-network-for-change-detection) | TensorFlow+Keras | Re3FCN | CNN (ConvLSTM); PCA; 3D convolution; Multi-class changes; Optical RS; Hyperspectral | 基于递归三维全卷积网络的高光谱图像变化检测,RS,2018. [paper](https://doi.org/10.3390/rs10111827)
[code, dataset](https://github.com/mkbensalah/Change-Detection-in-Hyperspectral-Images%20target=) | TensorFlow+Keras | FC-EF, FC-Siam-conc, FC-Siam-diff | CNN (UNet); Pure-Siamese; Optical RS | 用于变化检测的全卷积暹罗网络,ICIP,2018。 [paper](https://arxiv.org/abs/1810.08462)
[code, dataset](https://github.com/rcdaudt/fully_convolutional_change_detection) | Pytorch | CosimNet | CNN (Deeplab v2); Pure-Siamese; Streetview | 学习测量变化:用于场景变化检测的全卷积暹罗度量网络,arXiv,2018。 [paper](https://arxiv.org/abs/1810.09111)
[code, dataset, pre-trained model](https://github.com/gmayday1997/SceneChangeDet) | Pytorch+Python2.7 | Mask R-CNN | Mask R-CNN (ResNet-101); Transfer Learning; Post-Classification; Optical RS | Slum segmentation and change detection: a deep learning approach, NIPS, 2018. [paper](https://arxiv.org/abs/1811.07896)
[code, dataset, pre-trained model](https://github.com/cbsudux/Mumbai-slum-segmentation) | TensorFlow+Keras | CaffeNet | CNN (CaffeNet); Unsupervised; Transfer Learning; Optical RS | 基于卷积神经网络特征的卫星图像变化检测,IWPR,2016. [paper](https://doi.org/10.1117/12.2243798)
[code, dataset](https://github.com/vbhavank/Unstructured-change-detection-using-CNN) | TensorFlow+Keras | CWNN | CNN (CWNN); Unsupervised; Pre-Classification; SAR | 基于卷积小波神经网络的SAR图像海冰变化检测,GRSL,2019 [paper](https://dx.doi.org/10.1109/LGRS.2019.2895656)
[code, dataset](https://github.com/summitgao/SAR_Change_Detection_CWNN) | Matlab | MLFN | CNN (DenseNet); Transfer learning; SAR | 从合成孔径雷达图像进行海冰变化检测的转移深度学习,GRSL,2019。 [paper](https://dx.doi.org/10.1109/LGRS.2019.2906279)
[code, dataset](https://github.com/summitgao/SAR-Change-Detection-MLFN) | Caffe+Matlab | GarborPCANet | CNN (PCANet); Unsupervised; Pre-Classification; Gabor Wavelets; SAR | 基于PCANet的合成孔径雷达图像变化自动检测,GRSL,2016。 [paper](https://dx.doi.org/10.1109/LGRS.2016.2611001)
[code, dataset](https://github.com/summitgao/SAR_Change_Detection_GarborPCANet) | Matlab | Ms-CapsNet | CNN (Ms-CapsNet); Capsule; Attention Mechanism; Adaptive Fusion Convolution; SAR | 基于多尺度胶囊网络的SAR图像变化检测,GRSL,2020. [paper](https://dx.doi.org/10.1109/LGRS.2020.2977838)
[code, dataset](https://github.com/summitgao/SAR_CD_MS_CapsNet) | Matlab+Keras2.16 | DCNet | CNN; Unsupervised; Pre-Classification; SAR | 基于信道加权深度级联网络的合成孔径雷达图像变化检测,JSTARS,2019。 [paper](https://dx.doi.org/10.1109/JSTARS.2019.2953128)
[code, dataset](https://github.com/summitgao/SAR_CD_DCNet) | Caffe | ChangeNet | CNN; Siamese; StreetView | ChangeNet:用于视觉变化检测的深度学习架构,ECCV,2018。 [paper](http://openaccess.thecvf.com/content_eccv_2018_workshops/w7/html/Varghese_ChangeNet_A_Deep_Learning_Architecture_for_Visual_Change_Detection_ECCVW_2018_paper.html)
[code, dataset](https://github.com/leonardoaraujosantos/ChangeNet) | Pytorch | 2.2 传统方法的可用代码
表2。用于传统变化检测方法的可用代码列表。
方法 | 关键词 | 论文/代码 | 运行框架 | Several Classical Methods | CVA; DPCA; Image Differencing; Image Ratioing; Image Regression; IR-MAD; MAD; PCAkMeans; PCDA; KMeans; OTSU; Fixed Threshold | 遥感变化检测工具箱。 [code](https://github.com/Bobholamovic/ChangeDetectionToolbox) | Matlab | Matlab Toolbox Change Detection | IR-MAD; IT-PCA; ERM; ICM | 无监督变化检测分析工具箱,IJRS,2016.[paper](https://doi.org/10.1080/01431161.2016.1154226)
[code](https://github.com/NicolaFalco/Matlab-toolbox-change-detection) | Matlab | RFR,SVR,GPR | Unsupervised; Image Regression; Heterogeneous; Optical RS; SAR | 用于异构变化检测的无监督图像回归,TGRS,2019。 [paper](https://dx.doi.org/10.1109/TGRS.2019.2930348)
[code](https://github.com/llu025/Heterogeneous_CD/tree/master/legacy/Image_Regression) | Matlab | HPT | Unsupervised; Transformation; Heterogeneous; Optical RS; SAR | 通过同质像素变换在异质遥感图像中的变化检测,TIP,2018。 [paper](https://dx.doi.org/10.1109/TIP.2017.2784560)
[re-implementation code](https://github.com/llu025/Heterogeneous_CD/tree/master/legacy/Image_Regression) | Matlab | kCCA | Canonical Correlation Analysis; Cross-Sensor; Optical RS | 用自动核相关分析实现多时相跨传感器图像的光谱对准,IJPRS,2015。 [paper](https://doi.org/10.1016/j.isprsjprs.2015.02.005)
[code](https://sites.google.com/site/michelevolpiresearch/codes/cross-sensor) | Matlab | Ker. Diff. RBF | Unsupervised; K-means; Optical RS | 基于内核的无监督变化检测,GRSL,2012。 [paper](https://dx.doi.org/10.1016/j.jag.2011.10.013)
[code](https://drive.google.com/file/d/0B9xP9Y5JKJz0Q1ctbDJERWpTd2s/edit?usp=sharing) | Matlab | FDA-RM | DI-based; Frequency-Domain Analysis; Random Multigraphs; SAR | 基于频域分析和随机多图的合成孔径雷达图像变化检测,JARS,2018。 [paper](https://doi.org/10.1117/1.JRS.12.016010)
[code](https://github.com/summitgao/SAR_Change_Detection_FDA_RMG) | Matlab | CD-NR-ELM | DI-based; Pre-Classification; Extreme Learning Machine; SAR | 基于邻域比率和极限学习机的合成孔径雷达图像变化检测,JARS,2016。 [paper](https://doi.org/10.1117/1.JRS.10.046019)
[code, dataset](https://github.com/summitgao/SAR_Change_Detection_NR_ELM) | Matlab | None | Likelihood Ratio; Test Statistic; SAR | 极化SAR中的变化检测 影像,2015年。 [report](https://github.com/fouronnes/SAR-change-detection/blob/master/SAR_Change_Detection_Victor_Poughon.pdf)
[code](https://github.com/fouronnes/SAR-change-detection) | Python | PCA K-Means | Unsupervised; DI-based; PCA; K Means; Optical RS | 利用主成分分析和k-Means聚类的卫星图像无监督变化检测,GRSL,2009。 [paper](https://dx.doi.org/10.1109/LGRS.2009.2025059)
[re-implementation code, dataset](https://github.com/rulixiang/ChangeDetectionPCAKmeans) or [re-implementation code](https://github.com/leduckhai/Change-Detection-PCA-KMeans) | Matlab | PTCD | Tensor; Hyperspectral Optical RS | 用于无监督高光谱变化检测的三阶塔克分解重构检测器。乔家,2021年。 [paper](https://dx.doi.org/10.1109/JSTARS.2021.3088438)
[code, dataset](https://github.com/zephyrhours/Hyperspectral-Change-Detection-PTCD) | Matlab | GBF-CD | Data Fusion; Graph; EM; KI; | 基于图的数据融合在水稻作物变化检测和生物量估算中的应用。遥感,2020年 [paper](https://doi.org/10.3390/rs12172683)
[code, dataset](https://github.com/DavidJimenezS/GBF-CD) | Matlab | 3. 开放数据集
目前,已有一些用于变化检测的免费数据集,这些数据集可作为未来研究中AI训练和准确性评估的基准数据集。详细资料载于表3。
表3。用于更改检测的打开数据集列表。
类型 | 数据集名称 | 描述 | Optical RS | DSIFN Dataset [25](#Ref-25) | 6双时相高分辨率图像来自谷歌地球。有3600个图像对,大小为512×512,用于训练,340个用于验证,48个用于测试。 [Download](https://github.com/GeoZcx/A-deeply-supervised-image-fusion-network-for-change-detection-in-remote-sensing-images/tree/master/dataset) | Optical RS | S2MTCP [26](#Ref-26) | 1520幅 Sentinel-2 1C级图像对聚焦于全球城市地区,空间分辨率为10m,大小为600x600像素。不执行几何或辐射校正。 [Download](https://zenodo.org/record/4280482#.YQPtXI5LhjV) | Optical RS | SYSU-CD [27](#Ref-27) | 20000对2007年至2014年在香港拍摄的256×256的0.5米航空影像,包括6种变化类型:(a)新建城市建筑物;(b)郊区扩张;(c)施工前的基础工作;(d)植被的变化;(e)道路扩建;(f)海上建设。 [Download](https://github.com/liumency/SYSU-CD) | Optical RS | S2Looking [28](#Ref-28) | 建筑变化检测数据集由5000幅来自世界各地农村地区的配准双时态图像对(大小为1024*1024,0.5~0.8m/像素)和65,920多个标注的变化实例组成,分别表示新建和拆除的建筑[Download](https://github.com/AnonymousForACMMM/Dataset) | Optical RS | Synthetic and real images Dataset [29](#Ref-29) | 该数据库包含1.2万张无物体移位的三倍合成图像、1.2万张有物体移位的三倍模型图像和1.6万张真实遥感图像的三倍碎片。实验结果表明,所提出的CNN在合成图像和真实图像的变化检测中都是有效的 [Download](https://drive.google.com/file/d/1GX656JqqOyBi_Ef0w65kDGVto-nHrNs9) | Optical RS | SEmantic Change detectiON Dataset (SECOND) [24](#Ref-24) | 一个像素级标注的语义变化检测数据集,包括来自多个平台和传感器的4662对512×512像素的航空图像,覆盖杭州、成都和上海。它集中于6个主要的土地覆盖类别,即无植被地表、树木、低植被、水、建筑物和游乐场,它们经常涉及自然和人为的地理变化。[Download](http://www.captain-whu.com/PROJECT/SCD/) | Optical RS | Hyperspectral change detection dataset [1](#Ref-1) | 用AVIRIS和HYPERION传感器获得的3个不同的高光谱场景,分别为224和242个光谱波段,在像素水平上标记了5种与作物转换相关的变化类型. [Download](https://citius.usc.es/investigacion/datasets/hyperspectral-change-detection-dataset) | Optical RS | River HSIs dataset [2](#Ref-2) | 2 HSIs in Jiangsu province, China, with 198 bands, labeled as changed and unchanged at pixel level. [Download](https://drive.google.com/file/d/1cWy6KqE0rymSk5-ytqr7wM1yLMKLukfP/view) | Optical RS | HRSCD [3](#Ref-3) | 291对共同配准的RGB航拍影像,具有像素级变化和土地覆盖标注,提供分级级别变化标签,如1级标签包括无信息、人工地表、农业区、森林、湿地、水五类。 [Download](https://ieee-dataport.org/open-access/hrscd-high-resolution-semantic-change-detection-dataset) | Optical RS | WHU building dataset [4](#Ref-4) | 包含12,796栋建筑物的2期航空图像,以及建筑物矢量和栅格地图。 [Download](http://study.rsgis.whu.edu.cn/pages/download/) | Optical RS | SZTAKI Air change benchmark [5](#Ref-5), [6](#Ref-6) | 13幅1.5m空间分辨率航空影像对,在像素级标记为变化和不变. [Download](http://web.eee.sztaki.hu/remotesensing/airchange_benchmark.html) | Optical RS | OSCD [7](#Ref-7) | 利用Sentinel-2获取的24对多光谱图像,在像素水平标记为变化和不变。 [Download](https://rcdaudt.github.io/oscd/) | Optical RS | Change detection dataset [8](#Ref-8) | 4对不同空间分辨率的多光谱图像,在像素级标记为变化和不变。 [Download](https://github.com/MinZHANG-WHU/FDCNN) | Optical RS | MtS-WH [9](#Ref-9) | 2幅由IKONOS传感器采集的4个波段1 m空间分辨率的大尺寸VHR图像,在场景级标记了5种变化类型(停车区、稀疏房屋区、居民区和植被区) [Download](http://sigma.whu.edu.cn/newspage.php?q=2019_03_26) | Optical RS | ABCD [10](#Ref-10) | 16,950对RGB航空图像,用于探测被海啸冲刷的建筑物,并在现场级别标记受损建筑物。 [Download](https://github.com/gistairc/ABCDdataset) | Optical RS | xBD [11](#Ref-11) | 用于建筑物损害评估的灾前和灾后卫星图像,包括6种灾害类型的850,000多个建筑物多边形,在像素级标记4个损害等级。 [Download](https://xview2.org/dataset) | Optical RS | AICD [12](#Ref-12) | 1000对用渲染引擎生成的人工变化的合成航空图像,在像素级标记为变化和不变。 [Download](https://computervisiononline.com/dataset/1105138664) | Optical RS | Database of synthetic and real images [13](#Ref-13) | 由谷歌地球获得的24000幅合成图像和16000幅真实季节变化的遥感图像片段,在像素级别标记为变化和不变。 [Download](https://drive.google.com/file/d/1GX656JqqOyBi_Ef0w65kDGVto-nHrNs9/edit) | Optical RS | LEVIR-CD [14](#Ref-14) | 637个非常高分辨率(VHR,0.5米/像素)谷歌地球(GE)图像贴片对,大小为1024×1024像素,包含总共31,333个单独的变化建筑实例,在像素级别标记为变化和不变。 [Download](https://justchenhao.github.io/LEVIR/) | Optical RS | Bastrop fire dataset [21](#Ref-21) | 美国德克萨斯州巴斯特罗普县上空由不同传感器获得的图像。它由Landsat5 TM作为事件前的图像和Landsat5 TM、EO-1 ALI和Landsat8作为事件后的图像组成,在像素级标记为变化和不变,主要是由野火引起的。 [Download](https://sites.google.com/site/michelevolpiresearch/codes/cross-sensor) | Optical RS | Google data set [23](#Ref-23) | 19幅随季节变化的VHR图像配以红、绿、蓝3个波段,空间分辨率为0.55m,大小为1006×1168至4936×5224像素。形象变化包括水域、道路、农田、裸地、森林、建筑、船舶等,建筑构成主要变化。于2006年至2019年期间收购,覆盖中国广州市郊区。 [Download](https://github.com/daifeng2016/Change-Detection-Dataset-for-High-Resolution-Satellite-Imagery) | Optical RS & SAR | California dataset [22](#Ref-22) | 3幅图像,包括一幅由Landsat 8在2017年用9个通道捕获的遥感图像,一幅由Sentinel-1A在洪水发生后捕获的合成孔径雷达图像(以偏振度VV和VH记录),以及一幅地面真相图。 [Download](https://sites.google.com/view/luppino/data) | Optical RS & SAR | Homogeneous CD Dataset [30](#Ref-30) | 6种情景:情景1有两个单极化SAR数据集;有两个PolSAR数据集的方案2;具有两个光学图像数据集的场景3。异构OUSCD:两个SAR/光学(多光谱)数据集的场景4;情景5:从不同传感器获得两个不同波段的多光谱数据集;使用双极谱/光学(多光谱)数据集的情景6。 [Download](https://github.com/yulisun/INLPG) | Street view | VL-CMU-CD [15](#Ref-15) | 1362对共同配准的RGB和深度图像,在像素级标记地面真相变化(例如,垃圾箱、标志、车辆、垃圾、建筑、交通锥、人/自行车、障碍物)和天空掩码。 [Download](http://ghsi.github.io/proj/RSS2016.html) | Street view | PCD 2015 [16](#Ref-16) | 139/800 “海啸”和“GSV”子集中的200幅全景图像对,大小为224×1024像素,在像素级标记为变化和不变。 [Download](http://www.vision.is.tohoku.ac.jp/us/download/) | Street view | Change detection dataset [17](#Ref-17) | 车载摄像头在两个不同时间点拍摄的城市街道图像序列,大小为5000×2500像素,标记了像素级的三维场景结构变化。 [Download](http://www.vision.is.tohoku.ac.jp/us/research/4d_city_modeling/chg_dataset/) | CV | CDNet 2012 [18](#Ref-18) | 6个视频类别,每个类别4-6个视频序列,地面真理图像包含5个标签,即:静态、硬阴影、感兴趣区域外、未知运动(通常围绕运动物体,由于半透明和运动模糊)和运动。 [Download](http://jacarini.dinf.usherbrooke.ca/dataset2012/) | CV | CDNet 2014 [19](#Ref-19),[20](#Ref-20) | 6个视频类别,每个类别4-6个视频序列,地面真理图像包含5个标签,即:静态、硬阴影、感兴趣区域外、未知运动(通常围绕运动物体,由于半透明和运动模糊)和运动。 [Download](http://www.changedetection.net/) | CV | ChangeSim [31](#Ref-31) | 一个具有挑战性的数据集旨在在线场景变化检测和更多,在具有非目标环境变化的真实感模拟环境中收集数据,如空气浊度和光照条件变化,以及工业室内环境中目标对象的变化。 [Download](https://github.com/SAMMiCA/ChangeSim) | CV | 更多视频数据集 | 可以看出,可用于变更检测任务的开放数据集数量较少,其中一些数据大小较小。目前,仍缺乏可用于AI训练的大型SAR数据集。大多数基于人工智能的变化检测方法都是基于SAR数据集,这些数据集包括伯尔尼数据集、渥太华数据集、黄河数据集和墨西哥数据集,这些数据集包含的变化类型有限,无法满足复杂土地覆盖和变化类型多样的地区变化检测的需要。而且,它们的标签也不是免费提供的。在计算机视觉(CV)中,基于人工智能的变化检测方法通常采用街景数据集。在CV中,基于图片或视频的变化检测也是一个热门的研究领域,其基本思想与基于RS数据的变化检测是一致的。因此,除了街景图像数据集,CV中的几个视频数据集也可以用于基于AI的变化检测方法的研究,如CDNet 2012和CDNet 2014。
4. 应用程序
基于人工智能的变化检测技术的发展极大地方便了许多应用,提高了其自动化和智能化程度。大多数基于AI的变化检测生成二值映射,这些研究只关注算法本身,没有一个具体的应用领域。因此,可以认为它们一般适用于LULC变化检测。在本节中,我们将重点介绍与特定应用程序相关联的技术,它们可以大致分为四类: Urban contexts: 城市扩展、公共空间管理、建筑变化检测; Resources and environment:人为环境变化、水文环境变化、海冰、地表水和森林监测; Natural disasters: 滑坡制图与破坏评估; Astronomy: 行星表面。
我们概述了文献中针对不同应用类别的各种变化检测技术。表4列出了与这些应用程序相关联的工作和数据类型。
表4。基于AI的变化检测技术的主要应用综述。
应用 | 数据类型 | 相关论文 | Urban contexts | Urban expansion | Satellite images | Lyu et.al (2018), Tong et.al (2007) | SAR images | Iino et.al (2017) | Public space management | Street view images | Varghese et.al (2018) | Road surface | UAV images | Truong et.al (2020) | Building change detection | Aerial images | Ji et.al (2019), Sun et.al (2019), Nemoto et.al (2017) | Satellite images | Huang et.al (2019), Zhu et.al (2018) | Satellite/Aerial images | Jiang et.al (2020), Ji et.al (2018), Saha et.al (2020) | Airborne laser scanning data and aerial images | Zhang et.al (2019) | SAR images | Jaturapitpornchai et.al (2019) | Satellite images and GIS map | Ghaffarian et.al (2019) | Resources & environment | Human-driven environmental changes | Satellite images | Chen et.al (2016) | Hydro-environmental changes | Satellite images | Nourani et.al (2018) | Sea ice | SAR images | Gao et.al (2019), Gao et.al (2019) | Surface water | Satellite images | Song et.al (2019), Rokni et.al (2015) | Forest monitoring | Satellite images | Khan et.al (2017), Lindquist et.al (2016), Deilmai et.al (2014), Woodcock et.al (2001), Gopal et.al (1996) | Natural disasters | Landslide mapping | Aerial images | Fang et.al (2020), Lei et.al (2019) | Satellite images | Chen et.al (2018), Ding et.al (2016), Tarantino et.al (2006) | Damage assessment | Satellite images | caused by tsunami [Sublime et.al (2019)](https://dx.doi.org/10.3390/rs11091123),[Singh et.al (2015)](https://dx.doi.org/10.1007/s11069-015-1595-z), particular incident [Hedjam et.al (2019)](https://scholar.google.com/scholar_lookup?title=Change+detection+from+unlabeled+remote+sensing+images+using+siamese+ANN&conference=Proceedings+of+the+IGARSS+2019%E2%80%942019+IEEE+International+Geoscience+and+Remote+Sensing+Symposium&author=Hedjam,+R.&author=Abdesselam,+A.&author=Melgani,+F.&publication_year=2019&pages=1530%E2%80%931533), flood [Peng et.al (2019)](https://dx.doi.org/10.3390/rs11212492), or earthquake [Ji et.al (2019)](https://dx.doi.org/10.3390/rs11101202) | Aerial images | caused by tsunami [Fujita et.al (2017)](https://scholar.google.com/scholar_lookup?title=Damage+detection+from+aerial+images+via+convolutional+neural+networks&conference=Proceedings+of+the+2017+Fifteenth+IAPR+International+Conference+on+Machine+Vision+Applications+(MVA),+Nagoya+Univ&author=Fujita,+A.&author=Sakurada,+K.&author=Imaizumi,+T.&author=Ito,+R.&author=Hikosaka,+S.&author=Nakamura,+R.&publication_year=2017&pages=5%E2%80%938) | SAR images | caused by fires [Planinšič et.al (2018)](https://dx.doi.org/10.1109/LGRS.2017.2786344), or earthquake [Saha et.al (2018)](https://scholar.google.com/scholar_lookup?title=Destroyed-buildings+detection+from+VHR+SAR+images+using+deep+features&author=Saha,+S.&author=Bovolo,+F.&author=Bruzzone,+L.&publication_year=2018) | Street view images | caused by tsunami [Sakurada et.al (2015)](https://scholar.google.com/scholar_lookup?title=Change+detection+from+a+street+image+pair+using+CNN+features+and+superpixel+segmentation&conference=Proceedings+of+the+British+Machine+Vision+Conference+(BMVC)&author=Sakurada,+K.&author=Okatani,+T.&publication_year=2015&pages=61.1%E2%80%9361.12) | Street view images and GIS map | caused by tsunami [Sakurada et.al (2017)](https://dx.doi.org/10.1016/j.cviu.2017.01.012) | Astronomy | Planetary surfaces | Satellite images | Kerner et.al (2019) | 5. 软件程序
目前有大量带有变更检测工具的软件,我们对它们有一个简要的总结,见表5。
类型 | 名称 | 描述 | 商业 | ERDAS IMAGINE | 提供真实价值,将遥感、摄影测量、激光雷达分析、基本矢量分析和雷达处理整合为一个单一产品,包括多种 变化检测工具. | 商业 | ArcGIS | 使用 栅格计算器工具 或 深度学习工作流计算两个栅格数据集之间的变化检测. | 商业 | ENVI | 提供 变更检测分析工具 和 ENVI深度学习模块. | 商业 | eCognition | 可用于 各种变化映射, 通过利用谷歌TensorFlow™库的深度学习技术,eCognition为客户提供高度复杂的模式识别和相关工具,这些工具可以自动分类感兴趣的对象,以获得更快、更准确的结果,, 更多. | 商业 | PCI Geomatica | 提供 变化检测工具, 在许多需要分析变化的情况下都很有用,例如:风暴破坏、森林火灾破坏、洪水泛滥、城市蔓延 more. | 商业 | SenseTime | SenseRemote遥感智能解决方案 | 开源软件 | QGIS | 提供 检测工具. | 开源软件 | Orfeo ToolBox | 提供 多元变化检测器(MAD)算法. | 开源软件 | Change Detection ToolBox | 遥感变化检测的MATLAB工具箱. | 6. 遥感变化检测的相关论文推荐
以下论文有助于研究人员更好地理解遥感变化检测这一领域,见表6。
发布年份 | 查看论文 | 1989 | Digital change detection techniques using remotely sensed data, IJRS. [paper](https://dx.doi.org/10.1080/01431168908903939) | 2004 | Digital change detection methods in ecosystem monitoring: a review, IJRS. [paper](https://dx.doi.org/10.1080/0143116031000101675) | 2004 | Change detection techniques, IJRS. [paper](https://dx.doi.org/10.1080/0143116031000139863) | 2012 | Object-based change detection, IJRS. [paper](https://dx.doi.org/10.1080/01431161.2011.648285) | 2013 | Change detection from remotely sensed images: From pixel-based to object-based approaches, ISPRS. [paper](https://doi.org/10.1016/j.isprsjprs.2013.03.006) | 2016 | 3D change detection–approaches and applications, ISPRS. [paper](https://doi.org/10.1016/j.isprsjprs.2016.09.013) | 2016 | Deep learning for remote sensing data a technical tutorial on the state of the art, MGRS. [paper](https://dx.doi.org/10.1109/MGRS.2016.2540798) | 2017 | Comprehensive survey of deep learning in remote sensing: theories, tools, and challenges for the community, JRS. [paper](https://doi.org/10.1117/1.JRS.11.042609) | 2017 | Deep Learning in Remote Sensing, MGRS. [paper](https://dx.doi.org/10.1109/MGRS.2017.2762307) | 2018 | Computational intelligence in optical remote sensing image processing, ASOC. [paper](https://doi.org/10.1016/j.asoc.2017.11.045) | 2019 | A review of change detection in multitemporal hyperspectral images: current techniques, applications, and challenges, MGRS. [paper](https://dx.doi.org/10.1109/MGRS.2019.2898520) | 2019 | Deep learning in remote sensing applications: A meta-analysis and review, ISPRS. [paper](https://doi.org/10.1016/j.isprsjprs.2019.04.015) | 2020 | Deep Learning for change detection in remote sensing images: comprehensive review and meta-analysis, arXiv. [paper](https://arxiv.org/abs/2006.05612) | 2020 | Change detection based on artificial intelligence: state-of-the-art and challenges, RS. [paper](https://doi.org/10.3390/rs12101688) | 7. 参考资料
[1] Hyperspectral Change Detection Dataset. Available online: https://citius.usc.es/investigacion/datasets/hyperspectral-change-detection-dataset (accessed on 4 May 2020).
[2] Wang, Q.; Yuan, Z.; Du, Q.; Li, X. GETNET: A General End-to-End 2-D CNN Framework for Hyperspectral Image Change Detection. IEEE Trans. Geosci. Remote Sens. 2018, 57, 3–13. [Google Scholar] [CrossRef]
[3] Daudt, R.C.; Le Saux, B.; Boulch, A.; Gousseau, Y. Multitask learning for large-scale semantic change detection. Comput. Vis. Image Underst. 2019, 187, 102783. [Google Scholar] [CrossRef]
[4] Ji, S.; Wei, S.; Lu, M. Fully Convolutional Networks for Multisource Building Extraction from an Open Aerial and Satellite Imagery Data Set. IEEE Trans. Geosci. Remote Sens. 2018, 57, 574–586. [Google Scholar] [CrossRef]
[5] Benedek, C.; Sziranyi, T. Change Detection in Optical Aerial Images by a Multilayer Conditional Mixed Markov Model. IEEE Trans. Geosci. Remote Sens. 2009, 47, 3416–3430. [Google Scholar] [CrossRef]
[6] Benedek, C.; Sziranyi, T. A Mixed Markov model for change detection in aerial photos with large time differences. In Proceedings of the 2008 19th International Conference on Pattern Recognition, Tampa, FL, USA, 8–11 December 2008; pp. 1–4. [Google Scholar]
[7] Daudt, R.C.; Le Saux, B.; Boulch, A.; Gousseau, Y. Urban change detection for multispectral earth observation using convolutional neural networks. In Proceedings of the IGARSS 2018 IEEE International Geoscience and Remote Sensing Symposium, Valencia, Spain, 22–27 July 2018; pp. 2115–2118. [Google Scholar]
[8] Zhang, M.; Shi, W. A Feature Difference Convolutional Neural Network-Based Change Detection Method. IEEE Trans. Geosci. Remote Sens. 2020, 1–15. [Google Scholar] [CrossRef]
[9] Wu, C.; Zhang, L.; Zhang, L. A scene change detection framework for multi-temporal very high resolution remote sensing images. Signal Process. 2016, 124, 184–197. [Google Scholar] [CrossRef]
[10] Fujita, A.; Sakurada, K.; Imaizumi, T.; Ito, R.; Hikosaka, S.; Nakamura, R. Damage detection from aerial images via convolutional neural networks. In Proceedings of the 2017 Fifteenth IAPR International Conference on Machine Vision Applications (MVA), Nagoya Univ, Nagoya, Japan, 08–12 May 2017; pp. 5–8 [Google Scholar]
[11] Gupta, R.; Goodman, B.; Patel, N.; Hosfelt, R.; Sajeev, S.; Heim, E.; Doshi, J.; Lucas, K.; Choset, H.; Gaston, M. Creating xBD: A dataset for assessing building damage from satellite imagery. In Proceedings of the IEEE Conference on Computer Vision and Pattern Recognition Workshops, Long Beach, CA, USA, 16–20 June 2019; pp. 10–17. [Google Scholar]
[12] Bourdis, N.; Marraud, D.; Sahbi, H. Constrained optical flow for aerial image change detection. In Proceedings of the 2011 IEEE International Geoscience and Remote Sensing Symposium, Vancouver, BC, Canada, 24–29 July 2011; pp. 4176–4179. [Google Scholar] [CrossRef]
[13] Lebedev, M.A.; Vizilter, Y.V.; Vygolov, O.V.; Knyaz, V.A.; Rubis, A.Y. Change detection in remote sensing images using conditional adversarial networks. ISPRS Int. Arch. Photogramm. Remote Sens. Spat. Inf. Sci. 2018, 565–571. [Google Scholar] [CrossRef]
[14] Chen, H.; Shi, Z. A Spatial-Temporal Attention-Based Method and a New Dataset for Remote Sensing Image Change Detection. Remote Sensing, 12(10), 1662. [Google Scholar] [CrossRef]
[15] Alcantarilla, P.F.; Stent, S.; Ros, G.; Arroyo, R.; Gherardi, R. Street-view change detection with deconvolutional networks. Auton. Robot. 2018, 42, 1301–1322. [Google Scholar] [CrossRef]
[16] Sakurada, K.; Okatani, T. Change detection from a street image pair using CNN features and superpixel segmentation. In Proceedings of the British Machine Vision Conference (BMVC), Swansea, UK, 7–10 September 2015; pp. 61.1–61.12. [Google Scholar]
[17] Sakurada, K.; Okatani, T.; Deguchi, K. Detecting changes in 3D structure of a scene from multi-view images captured by a vehicle-mounted camera. In Proceedings of the IEEE Conference on Computer Vision and Pattern Recognition, Portland, OR, USA, 23–28 June 2013; pp. 137–144. [Google Scholar]
[18] Goyette, N.; Jodoin, P.-M.; Porikli, F.; Konrad, J.; Ishwar, P. Changedetection. net: A new change detection benchmark dataset. In Proceedings of the 2012 IEEE Computer Society Conference on Computer Vision and Pattern Recognition Workshops, Providence, RI, USA, 16–21 June 2012; pp. 1–8. [Google Scholar]
[19] Wang, Y.; Jodoin, P.-M.; Porikli, F.; Konrad, J.; Benezeth, Y.; Ishwar, P. CDnet 2014: An Expanded Change Detection Benchmark Dataset. In Proceedings of the 2014 IEEE Conference on Computer Vision and Pattern Recognition Workshops, Columbus, OH, USA, 23–28 June 2014; pp. 393–400. [Google Scholar]
[20] Goyette, N.; Jodoin, P.-M.; Porikli, F.; Konrad, J.; Ishwar, P. A Novel Video Dataset for Change Detection Benchmarking. IEEE Trans. Image Process. 2014, 23, 4663–4679. [Google Scholar] [CrossRef]
[21] Volpi, Michele; Camps-Valls, Gustau; Tuia, Devis (2015). Spectral alignment of multi-temporal cross-sensor images with automated kernel canonical correlation analysis; ISPRS Journal of Photogrammetry and Remote Sensing, vol. 107, pp. 50-63, 2015. [CrossRef]
[22] L. T. Luppino, F. M. Bianchi, G. Moser and S. N. Anfinsen. Unsupervised Image Regression for Heterogeneous Change Detection. IEEE Transactions on Geoscience and Remote Sensing. 2019, vol. 57, no. 12, pp. 9960-9975. [CrossRef]
[23] D. Peng, L. Bruzzone, Y. Zhang, H. Guan, H. Ding and X. Huang, SemiCDNet: A Semisupervised Convolutional Neural Network for Change Detection in High Resolution Remote-Sensing Images. IEEE Transactions on Geoscience and Remote Sensing. 2020. [CrossRef]
[24] Yang, Kunping, et al. Asymmetric Siamese Networks for Semantic Change Detection. arXiv preprint arXiv:2010.05687 (2020). [CrossRef]
[25] Zhang, C., Yue, P., Tapete, D., Jiang, L., Shangguan, B., Huang, L., & Liu, G. A deeply supervised image fusion network for change detection in high resolution bi-temporal remote sensing images. ISPRS Journal of Photogrammetry and Remote Sensing. 2020. [CrossRef]
[26] LEENSTRA, Marrit, et al. Self-supervised pre-training enhances change detection in Sentinel-2 imagery. arXiv. 2021. [CrossRef]
[27] SHI, Qian, et al. A Deeply Supervised Attention Metric-Based Network and an Open Aerial Image Dataset for Remote Sensing Change Detection. IEEE Transactions on Geoscience and Remote Sensing. 2021. [CrossRef]
[28] SHEN, Li, et al. S2Looking: A Satellite Side-Looking Dataset for Building Change Detection. arXiv. 2021. [CrossRef]
[29] LEBEDEV, M. A., et al. CHANGE DETECTION IN REMOTE SENSING IMAGES USING CONDITIONAL ADVERSARIAL NETWORKS. International Archives of the Photogrammetry, Remote Sensing & Spatial Information Sciences, 2018. [CrossRef]
[30] SUN, Yuli, et al. Structure Consistency-Based Graph for Unsupervised Change Detection With Homogeneous and Heterogeneous Remote Sensing Images. IEEE Transactions on Geoscience and Remote Sensing, 2021. [CrossRef]
[31] PARK, Jin-Man, et al. ChangeSim: Towards End-to-End Online Scene Change Detection in Industrial Indoor Environments. arXiv. 2021. [CrossRef] |
|